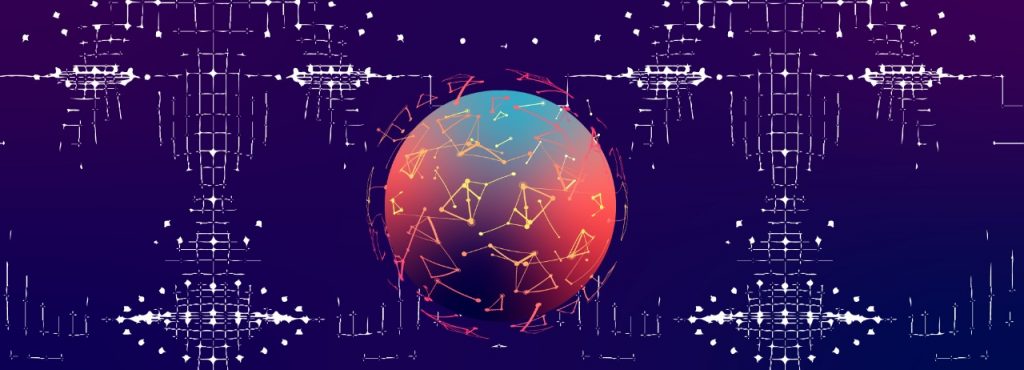
This blog series considers how biases and prejudices reflect online. It is often argued that the digital space fortifies prejudices against minority and marginalised groups online. The bias in online spaces are strongly connected to the inherent framing of internet and algorithms as well as the continued use of it by certain group of ‘privileged’ actors, who exert a power position not only to influence how the internet must work but also to impress upon the narratives created on the internet. These biases, commonly called “algorithmic biases”, have come to influence our lives as we increasingly operate between the fluidity of online and offline spaces.
An algorithm is a technique in which a computer is given a set of instructions and trained to perform a specific task or solve a problem in a particular way. In other words, an algorithm is trained what to do and how to do it. Such an operation includes transforming the input of data by processing it into useful output for the problem. By employing algorithms to analyse the data input and provide results by relying on the instructions it has been trained with, we allow it to make decisions on our behalf. As a result, these decisions are made without any human interference and while algorithmic mechanisms are deemed to be objective and unbiased, they have proven to be just as vulnerable to biases as humans. Safiya Noble, the author of Algorithms of Oppressions, argues that the algorithmic bias is a result of an unregulated internet which has biases like sexism and racism built into its architecture, design and language. Noble emphasises that the absence of human and social context in algorithmically driven decision-making processes adversely impacts marginalised groups and replicates their oppression from an offline to an online world.
The path to holding algorithms accountable begins with acknowledging the unregulated nature of the internet and the part it plays in algorithmic discrimination. Internet platforms are private players, and therefore, when their algorithms present “glitches” there is little to no accountability as to how they are fixed. For example, Google face recognition and auto-tagging algorithms tagged the faces of African American persons as ‘apes’ or ‘animals’. After a series of such incidents in which a bias against a certain protected group was publicly noted and condemned, a Google spokesperson told the media that Google is “working on the issue”. However, there can be no certainty that problems of this nature were solved or not.
This issue of algorithmic discrimination and accountability is not just limited to the internet. Similar inconsistencies are also seen in law enforcement algorithms: ‘Predpol’, an algorithm to forecast when and where crime will occur, with the goal of reducing human bias in policing, in fact repeatedly led police officers to target certain racial minorities, since the data input of the algorithm relied on reports by the police and not the actual crime rate of a particular neighbourhood. As a result, the algorithm reproduced the bias of the police officers.
Similarly, in the Netherlands, the Dutch government relied on an algorithm to determine which families had committed tax fraud, The algorithm flagged families based on stereotypical biases such as foreign origin and ‘foreign sounding’ names, forcing them to pay back thousands of euros of allowances. This led to the resignation of the Dutch cabinet when the scandal came to light. The prevalence of such incidents is regular both in public and private spheres.
Algorithms are in the business of deciding which neighbourhoods get policed, which persons are suspects for a crime, who gets censored on the internet and who gets recruited etc. The extent of such power and a lack of adequate regulation at the algorithmic side of things is proving harmful for those from protected groups. For example, a faulty face recognition algorithm led to Robert Williams, a Black man, being falsely arrested since his face was misidentified by the algorithm.
In the United States, the New York Civil Liberties Union filed a class-action suit against the Immigration and Customs Enforcement (ICE) for rigging their detention and release algorithm. The agency relied on a risk clarification algorithm to determine whether an arrested immigrant should be released or kept back 48 hours after being detained, while their case goes on in court. The algorithm took into account family ties, years in country and other such factors. No risk or low risk immigrants were supposed to be released. Until 2017, about half of those arrested for civil immigration offenses and deemed low risk were usually released. However, after 2017, 97% immigrants were detained and almost none were released. Furthermore, detainees had no information as to how they were classified by the algorithm nor did they have access to a lawyer. In 2018, Reuters reported that the algorithm was edited to eliminate the “release” option for immigrants. Such detention, by relying on an algorithm, highlights the power of the algorithm in our lives and systems and are also a reminder of the threat they pose to constitutional guarantees of due process. Such an example also urges us to determine our constitutional rights and guarantees in light of the rise in algorithmic usage and how they can be accountable to us. Another case that highlights this is Houston Federation of Teachers et al. v. Houston ISD. In the present case, some teachers had been fired on the basis of low scores on a teaching accountability algorithm. The judge held that they had been deprived of their property interest in employment and their due process rights had been violated because the algorithm was treated as a trade secret and could not reveal to the teachers on what basis low scores had been accorded to them. Eventually, the district of Houston settled with its teachers. Nevertheless, the case becomes an examples of how algorithms can fail to be accountable to users and become a threat to constitutional rights.
While private actors are making a lot of noise towards eliminating algorithmic bias by hiring diverse data teams, offering perspective when creating algorithms, and incorporating bias-trainings etc., the progress towards achieving algorithmic impartiality is excruciatingly slow.
The awareness that deep prejudices and biases could be reproduced and embedded in code urges us to carefully curate systems and policies that would inhibit such incidents. It also encourages us to closely introspect how we let our social inequalities affect the automated decision-making systems that largely influence many lives. In such a context, using intersectionality not only as a working framework to understand online biases but also as a basis to create policy and eradicate such bias is a step forward and will be explored in a future blog.
—————
This blog is first in the series of conversation on digital futures by Foundation London Story.
Author(s):
- N. Nagpal is a research intern at the Foundation London Story
- Dr. R. Manuvie is a Lecturer of Law at the University of Groningen